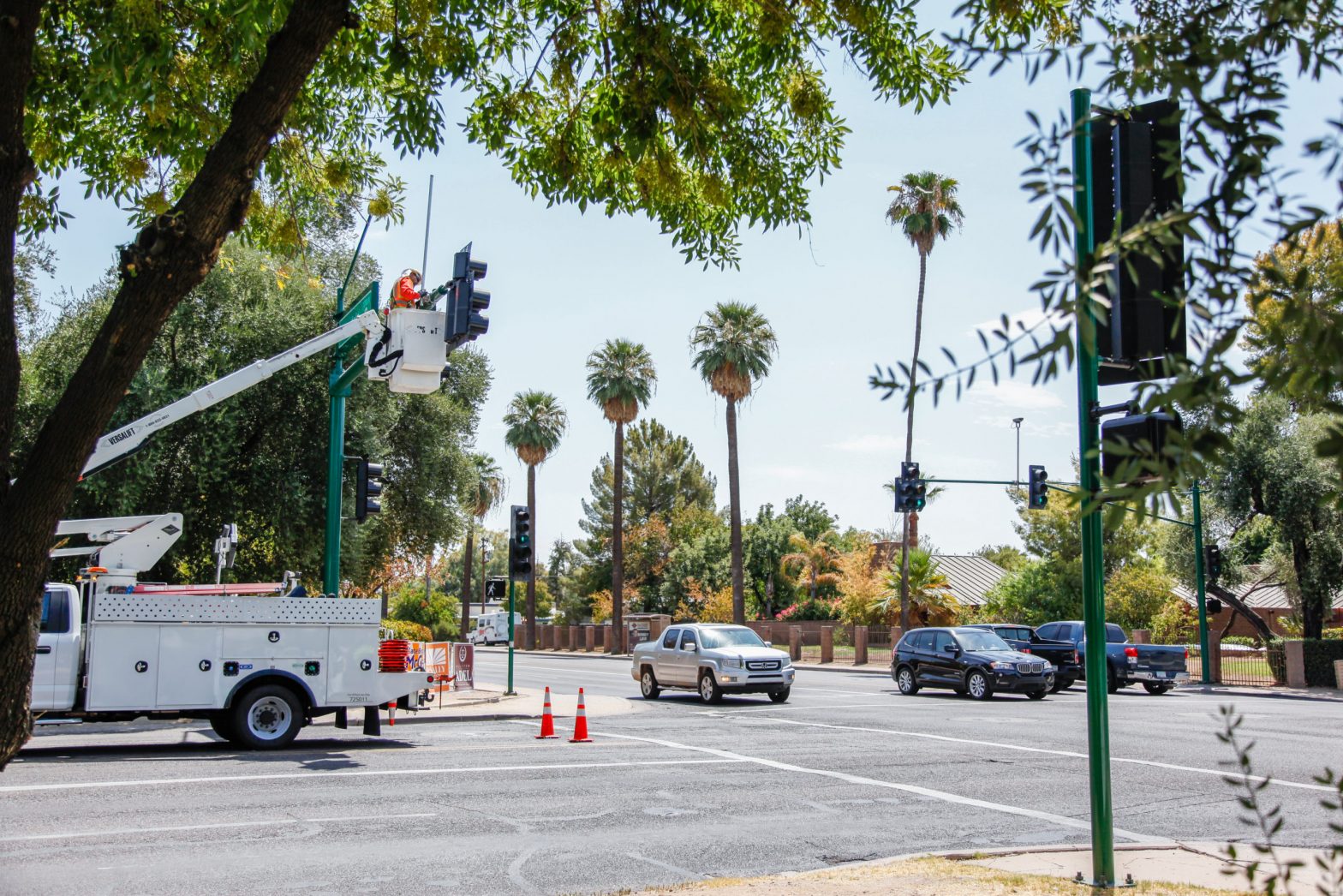
Artificial Intelligence Based Cloud Computing for Autonomous Traffic Management
Automobile-related deaths rank as one of the most common causes of death in many places, particularly developing countries; Egypt loses about 12,000 lives due to road traffic crashes every year. The greatest danger to human beings is not cars but people themselves because cars are not dangerous if driven by care and more attention. Cell phone use, whether by talking on the phone or texting, represents 25% of all automobile accidents, and 33% of human error accidents occur due to diseases associated with sleep disorders. Therefore, if the driver gets a warning 1.5 seconds earlier before the collision occurs, 80% of roadway accidents will be reduced, and cars will have the ability to make emergency breaks in time. In this project, we propose a cloud-based AI algorithm to anticipate the detection and localization of road hazards to keep the road safe. The main objectives are to avoid collision between vehicles and manage road traffic. Also, cloud-based AI computing will achieve the expected challenges: high vehicle mobility, minimal latency, and real-time services.
Objective/Contributions:
- An in-depth understanding of the current AI-based traffic control for autonomous vehicles solutions.
- Develop a cloud-based AI algorithm that can perform real-time analysis of data collected from autonomous cars to meet the latency requirements.
- Develop a data fusion-based solution and resource management algorithm with AI that helps in enhancing decision-making capabilities to avoid the collision.
- Three high-quality scientific articles are to be published.
Outcome Publications:
- Retaj Yousri, Mustafa A. Elattar, and M. Saeed Darweesh, “A Deep Learning-Based Benchmarking Framework for Lane Segmentation in the Complex and Dynamic Road Scenes,” in IEEE Access, vol. 9, pp. 117565-117580, 2021, DOI: 10.1109/ACCESS.2021.3106377.
- Retaj Yousri, Mohamed Mostafa, Rawane Yasser, Mahmoud Shawki, Ahmed Khaled, Ziad Mostafa, Ahmed Soltan, and M. Saeed Darweesh, “A Real-Time Approach Based on Deep Learning for Ego-Lane Detection,” in International Japan-Africa Conference on Electronics, Communications, and Computations (JAC-ECC-2021), Alexandria, Egypt, December 2021.